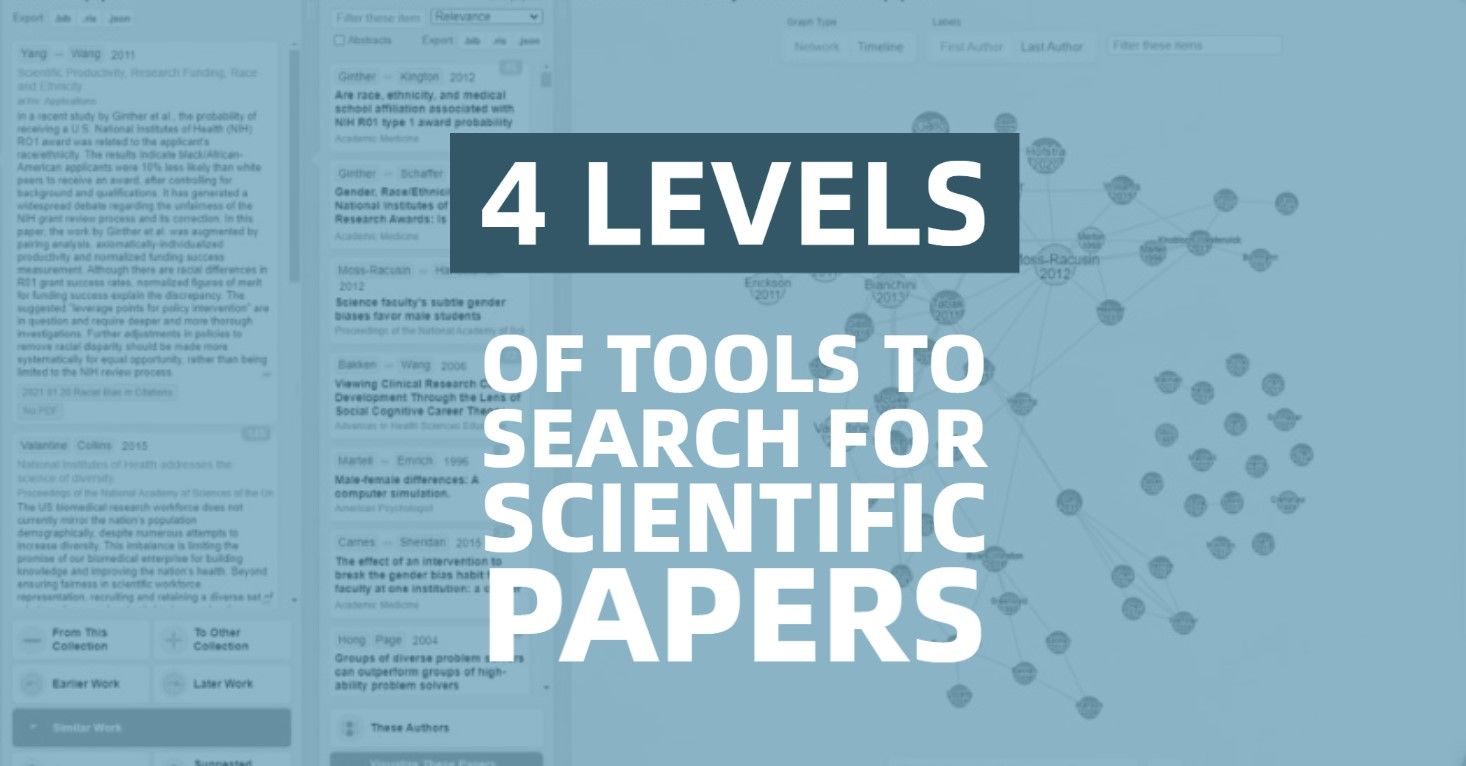
4 levels
From our personal experience, there are currently 4 levels of tools. We categorize them based on which part of the paper (or its metadata) that is searched, and the output they are able to provide: ranking, relation, data extraction
- Rank papers, based on word matching in title & keywords— Cible+, Scopus, PubMed, Google Scholar, Science Direct, Web of Science, etc
- Map network of citations— Connected Papers, Rabbit Research, Open Knowledge Map
- Provides summary, influence-in-literature metrics, and rank papers, based on abstract and paper — Semantic Scholar
- Extract & creates a table of information based on the AI-reading of the abstract and paper — Elicit
Note that all methods use the title, keywords, and citation count.
In most cases, we can’t explain the methods of each toos, either because they are not disclosed, (eg), or is too complex and beyond our scope.